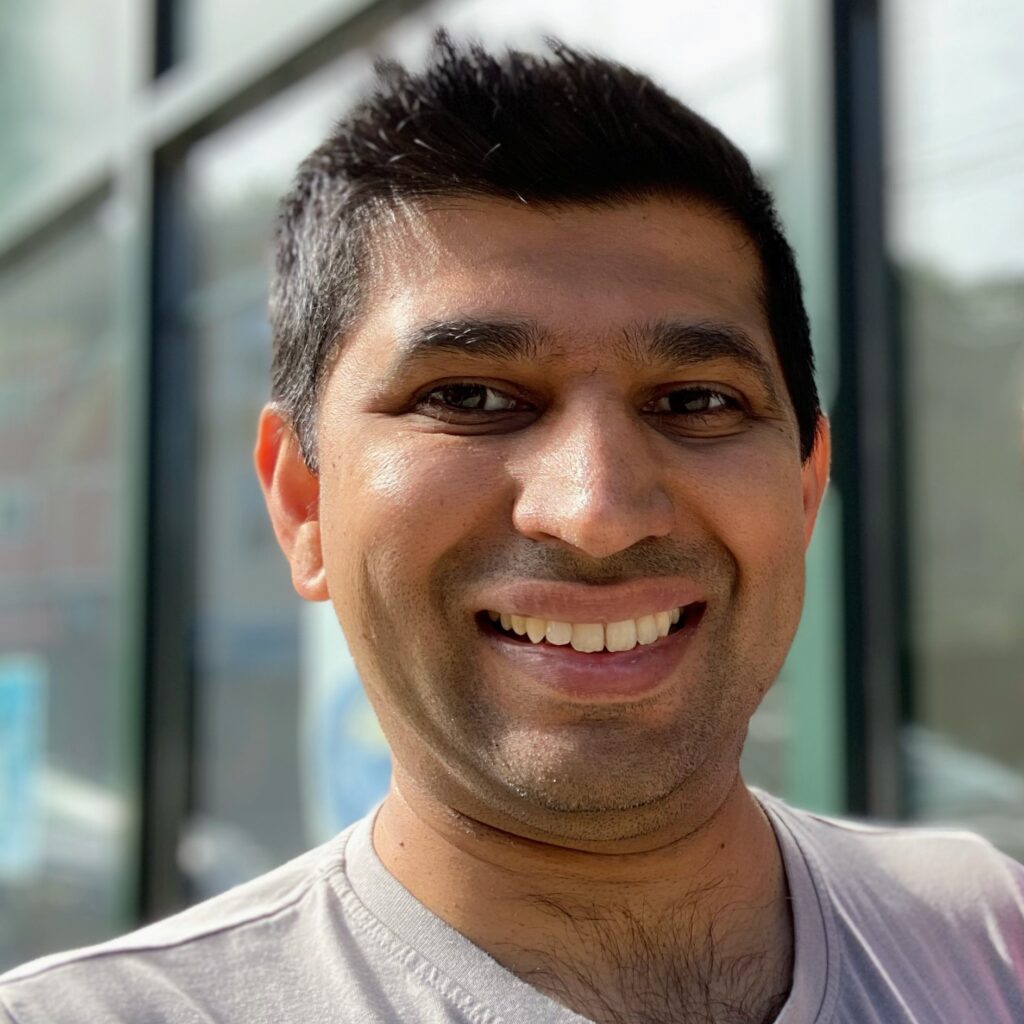
I am Srikanth Sastry. I am currently an engineering lead at Meta working on privacy infrastructure. Before joining Facebook, I was a software engineer at Google in Cambridge, MA, and before that I was a postdoctoral associate with Nancy Lynch at CSAIL, MIT. Prior to that, I received my PhD from Texas A&M University.
My professional interests include distributed system design, software engineering processes, and building engineering teams.
In a previous life, during my years in academia, I worked on distributed systems, networking, fault tolerance, and algorithm design and analysis. My list of publications are available on DBLP.
You can contact me on any of these channels: